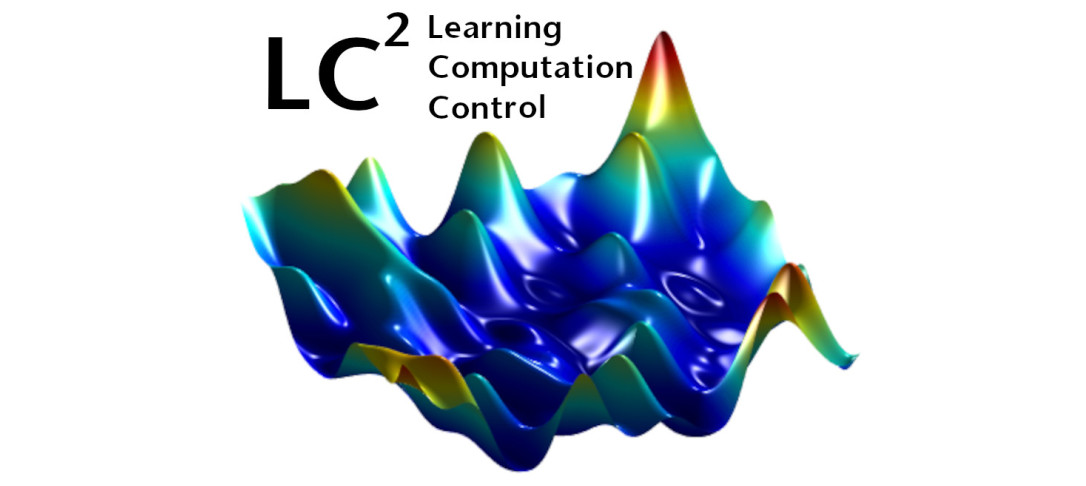
Denis Ullmo: Mean Field Game Approach to Non-Pharmaceutical Interventions in a Social Structure model of Epidemics
The design of coherent and efficient policies to address infectious diseases and their consequences requires to model not only epidemics dynamics, but also individual behaviors, as the latter has a strong influence on the former. Here, I will introduce a theoretical model for this problem, taking into account the social structure of a population. This model is based on a Mean Field Game version of a SIR compartmental model, in which individuals are grouped by their age class and interact together in different settings. This social heterogeneity allows to reproduce realistic situations while remaining usable in practice. In this game theoretical approach, individuals can choose to limit their contacts by making a trade-off between the risks incurred by infection and the cost of being confined. The aggregation of all these individual choices and optimizations forms a Nash equilibrium through a system of coupled equations that we derive and solve numerically.
The global cost born by the population within this scenario is then compared to its societal optimum counterpart (i.e.\ the cost associated with the optimal set of strategies from the point of view of the society as a whole), and we investigate how the gap between these two costs can be partially bridged within a constrained Nash equilibrium for which a governmental institution would, under specific conditions, impose “partial lock-downs” such as the ones that were imposed during the Covid-19 pandemic.
Finally, I will consider the consequences of the finiteness of the population size N, or of a time T at which an external event (e.g.\ a vaccine) would end the epidemic, and show that the variation of these parameters could lead to “first order phase transitions” in the choice of optimal strategies.
Nathan Kutz: Modern Sensing and Learning with Machine Learning
Sensing is a universal task in science and engineering. Downstream tasks from sensing include learning dynamical models, inferring full state estimates of a system (system identification), control decisions, and forecasting. These tasks are exceptionally challenging to achieve with limited sensors, noisy measurements, and corrupt or missing data. Existing techniques typically use current (static) sensor measurements to perform such tasks and require principled sensor placement or an abundance of randomly placed sensors. In contrast, we propose a SHallow REcurrent Decoder (SHRED) neural network structure which incorporates (i) a recurrent neural network (LSTM) to learn a latent representation of the temporal dynamics of the sensors, and (ii) a shallow decoder that learns a mapping between this latent representation and the high-dimensional state space. By explicitly accounting for the time-history, or trajectory, of the sensor measurements, SHRED enables accurate reconstructions with far fewer sensors, outperforms existing techniques when more measurements are available, and is agnostic towards sensor placement. In addition, a compressed representation of the high-dimensional state is directly obtained from sensor measurements, which provides an on-the-fly compression for modeling physical and engineering systems. Forecasting is also achieved from the sensor time-series data alone, producing an efficient paradigm for predicting temporal evolution with an exceptionally limited number of sensors. In the example cases explored, including turbulent flows, complex spatio-temporal dynamics can be characterized with exceedingly limited sensors that can be randomly placed with minimal loss of performance.